- Bits of Brilliance
- Posts
- Brains Aren’t Just Big Neural Nets
Brains Aren’t Just Big Neural Nets
AIs understand without any understanding
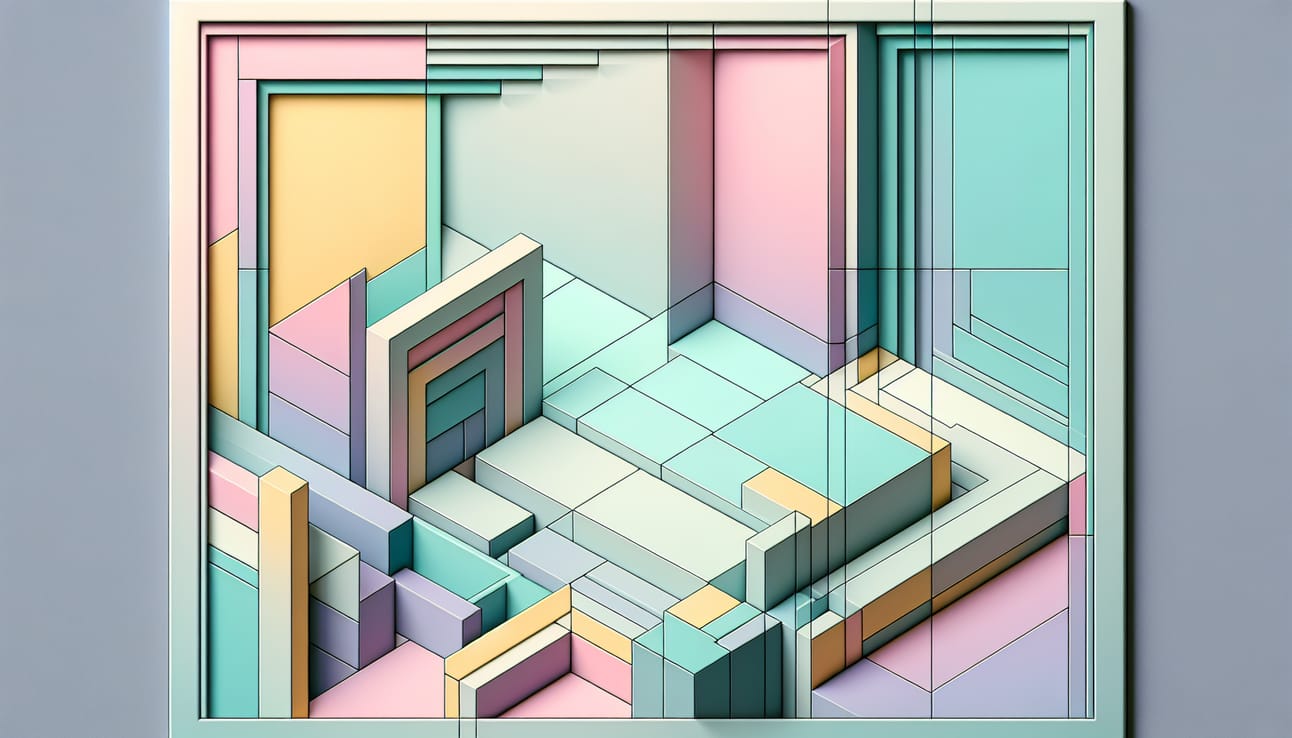
The first people who tried to build thinking machines thought it would be easy. Brains are made of neurons, neurons are simple, so if you wire up enough of them you’ll get intelligence. That was the idea behind McCulloch and Pitts’s early models: treat neurons as logic gates, stack them up, and watch thought emerge.
Decades later, we’ve built artificial neural networks with billions of parameters. They can recognize cats, translate languages, and play games better than any human. But for all their power, they’re still missing something essential. They don’t understand what they’re doing. They don’t know what a cat is, or what it means to win a game. They’re not even aware they exist.
Why? The problem is that the analogy between brains and neural nets was always superficial. Brains aren’t just a lot of simple units wired together. They’re the product of hundreds of millions of years of evolution, packed with specialized circuits designed for survival in a chaotic world. Neurons in brains don’t fire in neat layers; they form loops, feedback on themselves, and constantly interact with the body and the environment.
Brains are embedded in bodies. They get a constant stream of feedback from muscles, eyes, skin, and gut. Intelligence, for us, is tangled up with having a body and living in the world. Most artificial neural nets are nothing like this. They’re detached from reality, trained on static datasets, and never see the consequences of their actions. It’s the difference between looking at a map of a city and actually living in it. By all accounts, linguists would have never achieved machine translation without giving up on the central premises they had long focused on—the machine doesn’t actually need to know what a natural language is or how it works to create with it.
A child can learn what a dog is after meeting just a few of them—seeing, touching, maybe getting licked. An artificial neural net needs thousands or millions of labeled pictures. Even then, it doesn’t know what a dog smells like, or what happens if you pull its tail. It will report out notions of what might be the case but those are not understood in any sense of the word. Brains generalize from sparse, rich experience. Neural nets need brute force. The difference isn’t just data; it’s the kind of experience.
Some researchers think the gap will close if we just make bigger models, or build hardware that works more like real neurons. Maybe. But so far, more scale hasn’t led to understanding. GPT-4.1 (in Spring 2025) can write poetry, but it doesn’t know it’s writing. AlphaGo can win at Go, but it has no idea what a game is. Human intelligence is shaped by the fact that we have bodies, make mistakes, pursue goals, and care about outcomes. Until AI can do those things, it will remain a very clever tool, not a mind. And some predict that the next generation of AI will be defined by those experiences.
But maybe the goal isn’t to copy the brain exactly. Airplanes don’t flap their wings like birds, but they still fly—because they borrow the key idea of lift. Maybe what we need is to find the deeper principles behind intelligence: learning from experience, grounding ideas in the world, pursuing goals. We can be inspired by biology without being limited by it.
This isn’t just a technical question. It forces us to ask what intelligence really is. Is it just computation, or is it something that can only emerge from a body living in a world? If we keep chasing artificial intelligence, we might end up learning more about our own minds than we expected. Indeed, trying to build a brain out of silicon has led us to bigger questions: What does it mean to understand? What does it mean to be embodied? In the end, the value may not be in building a perfect artificial brain, but in forcing ourselves to look harder at what intelligence really requires. Sometimes, the most useful thing about chasing an answer is the new questions it makes us ask.
Reply